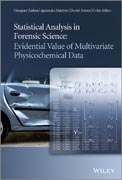
Statistical Analysis in Forensic Science
Zadora, Grzegorz
Martyna, Agnieszka
Ramos, Daniel
Aitken, Colin G. ,Dr
A practical guide for determining the evidential value of physicochemical data Microtraces of various materials (e.g. glass, paint, fibres, and petroleum products) are routinely subjected to physicochemical examination by forensic experts, whose role is to evaluate such physicochemical data in the context of the prosecution and defence propositions. Such examinations return various kinds of information, including quantitative data. From the forensic point of view, the most suitable way to evaluate evidence is the likelihood ratio. This book provides a collection of recent approaches to the determination of likelihood ratios and describes suitable software, with documentation and examples of their use in practice. The statistical computing and graphics software environment R , pre–computed Bayesian networks using Hugin Researcher and a new package, calcuLatoR , for the computation of likelihood ratios are all explored. Statistical Analysis in Forensic Science will provide an invaluable practical guide for forensic experts and practitioners, forensic statisticians, analytical chemists, and chemometricians. Key features include: Description of the physicochemical analysis of forensic trace evidence. Detailed description of likelihood ratio models for determining the evidential value of multivariate physicochemical data. Detailed description of methods, such as empirical cross–entropy plots, for assessing the performance of likelihood ratio–based methods for evidence evaluation. Routines written using the open–source R software, as well as Hugin Researcher and calcuLatoR . Practical examples and recommendations for the use of all these methods in practice. INDICE: Preface 1 Physicochemical data obtained in forensic science laboratories 1.1 Introduction 1.2 Glass 1.2.1 SEM–EDX technique 1.2.2 GRIM technique 1.3 Flammable liquids – ATD–GC/MS technique 1.4 Car paints – Py–GC/MS technique 1.5 Fibres and inks – MSP–DAD technique References 2 Evaluation of evidence in the form of physicochemical data 2.1 Introduction 2.2 Comparison problem 2.2.1 Two–stage approach 2.2.2 Likelihood ratio approach 2.2.3 Difference between an application of two–stage approach and likelihood ratio approach 2.3 Classification problem 2.3.1 Chemometric approach 2.3.2 Likelihood ratio approach 2.4 Likelihood ratio as a part of Bayes theory References 3 Continuous data 3.1 Introduction 3.2 Data transformations 3.3 Descriptive statistics 3.3.1 Measures of location 3.3.2 Dispersion – variance estimation 3.3.3 Data Distribution 3.3.4 Correlation 3.3.5 Continuous probability distributions 3.4 Hypothesis testing 3.4.1 Introduction 3.4.2 Hypothesis test for a population mean for samples with known variance σ 2 from a normal distribution 3.4.3 Hypothesis test for a population mean for small samples with unknown variance σ 2 from a normal distribution 3.4.4 Relation between tests and confidence intervals 3.4.5 Hypothesis test based on small samples for a difference in the means of two independent populations with unknown variances from normal distributions 3.4.6 Paired comparisons 3.4.7 Hotelling’s T 2 test 3.4.8 Significance test for correlation coefficient 3.5 Analysis of variance 3.5.1 Principles of ANOVA 3.5.2 Feature selection with application of ANOVA 3.5.3 Testing of the equality of variances 3.6 Cluster Analysis 3.6.1 Similarity measurements 3.6.2 Hierarchical Cluster Analysis 3.7 Dimensionality reduction 3.7.1 Principal Component Analysis 3.7.2 Graphical Models References 4 Likelihood ratio models for comparison problem 4.1 Introduction 4.2 LR model which assumes a normal between–object distribution 4.2.1 LR model for multivariate data 4.2.2 LR model for univariate data 4.3 LR models when between–object distribution is modelled by a kernel density estimation procedure 4.3.1 LR model for multivariate data 4.3.2 LR model for univariate data 4.4 Examples of application 4.4.1 Univariate data – LR model under assumption of normal between object distribution. Application of R code in research studies 1 4.4.2 Univariate data – LR model under assumption of normal between object distribution. Application of Bayesian Network model in casework studies 4.4.3 Univariate data – LR model which applies the kernel density estimation procedure. Application of R software in research studies 4.4.4 Univariate data – LR model, which applies kernel density estimation procedure. Application of calcuLatoR software in casework studies 4.4.5 Multivariate data – LR model under assumption of normal between object distribution. Application of R software in research studies 4.4.6 Multivariate data – LR model which applies the kernel density estimation procedure. Application of R software in research studies 4.4.7 Multivariate data – LR model which applies the kernel density estimation procedure. Application of R software in casework studies 4.5 R Software 4.5.1 Routines for casework application 4.5.2 Routines for research application References 5 Likelihood ratio models for classification problem 5.1 Introduction 5.2 LR model which assumes a normal between–object distribution 5.2.1 LR model for multivariate data 5.2.2 LR model for univariate data 5.2.3 One level models 5.3 LR model when between–object distribution is modelled by a kernel density estimation procedure 5.3.1 LR model for multivariate data 5.3.2 LR model for univariate data 5.3.3 One level models 5.4 Examples of the application 5.4.1 Univariate data – LR model under assumption of a normal between object distribution. Application of the Bayesian Network model in casework studies 5.4.2 Univariate data – LR model which applies the kernel density estimation procedure. Application of R software in research studies 5.4.3 Multivariate data – LR model which applies the kernel density estimation procedure. Application of R software in research studies 5.4.4 Multivariate data – LR model which applies the kernel density estimation procedure. Application of R software in casework studies 5.5 R software 5.5.1 Routines for casework application 5.5.2 Routines for research application References 6 Performance of likelihood ratio methods 6.1 Introduction 6.2 Empirical measurement of the performance of likelihood ratios 6.3 Histograms and Tippett plots 6.4 Measuring discriminating power 6.4.1 False positive and false negative rates 6.4.2 Discriminating power: a definition 6.4.3 Measuring discriminating power with DET curves 6.4.4 Is discriminating power enough? 6.5 Accuracy equals discriminating power plus calibration: Empirical Cross– Entropy plots 6.5.1 Accuracy in a classical example: weather forecasting 6.5.2 Calibration 6.5.3 Adaptation to forensic inference using likelihood ratios 6.6 Comparison of the performance of different methods for LR computation 6.6.1 MSP–DAD data from comparison of inks 6.6.2 Py–GC/MS data from comparison of car paints 6.6.3 SEM–EDX data for classification of glass objects 6.7 Conclusions: what to measure, and how? 6.8 Software – R code References Appendix A Probability A.1 Laws of probability A.2 Bayes theory and likelihood ratio A.3 Probability distributions for discrete data A.4 Probability distributions for continuous data References Appendix B Matrices and vectors – an introduction to the matrix algebra B.1 Operations on matrices B.1.1 Multiplication by a constant B.1.2 Adding matrices B.1.3 Multiplying matrices B.1.4 Matrix transposition B.1.5 Determinant of the matrix B.1.6 Matrix inversion B.1.7 Matrix equations B.1.8 Eigenvectors and eigenvalues References Appendix C Pool Adjacent Violators Algorithm References Appendix D Introduction to R software D.1 Becoming familiar with R D.2 Basic mathematical operations in R D.2.1 Vector algebra D.2.2 Matrix algebra D.3 Data insertion D.4 Functions in R D.5 Dereferencing D.6 Basic statistical functions D.7 Graphics with R D.7.1 Box–plots D.7.2 Q–Q plots D.7.3 Normal distribution D.7.4 Histograms D.7.5 Kernel Density Estimation D.7.6 Correlation between variables D.8 Data saving D.9 Casework application in comparison problem – codes D.9.1 Function UC saved in a file UC—comparison—calculations.R D.9.2 Function LR.Nor.function saved in a file LR—comparison—Nor.R D.9.3 Function LR.KDE.function saved in a file LR—comparison—KDE.R D.9.4 The code used for casework application available in comparison—casework—code.R D.10 Research application in comparison problem – codes D.10.1 Code for the level of false answers rates establishment available in comparison—research—code.R D.11 Casework application in classification problem – codes D.11.1 Function UC.1 for two–level model available in a file UC1—two—level—calculations.R D.11.2 Function LR.Nor.function available in a file LR—classification—two—level—Nor.R D.11.3 Function LR.KDE.function available in a file LR—classification—two—level—KDE.R D.11.4 The code classification—casework—code.R used for casework application D.12 Research application in classification problem – codes D.12.1 The code for classification problem is given below and is available in classification—research—code.txt D.13 Evaluating the performance of the LR models – codes for histograms, DET, Tippett, and ECE plots D.13.1 Histograms D.13.2 Tippett plots D.13.3 DET plots D.13.4 ECE plots References Appendix E Bayesian Networks models E.1 Introduction to Bayesian Networks E.2 Introduction to HuginTMSoftware E.2.1 HuginTM– basic functions E.2.2 A creation of a new Bayesian Network E.2.3 Performance of calculations References Appendix F Introduction to CalcuLatoR software F.1 Introduction F.2 Manual References Index
- ISBN: 978-0-470-97210-6
- Editorial: Wiley–Blackwell
- Encuadernacion: Cartoné
- Páginas: 336
- Fecha Publicación: 10/01/2014
- Nº Volúmenes: 1
- Idioma: Inglés